Artificial intelligence, computing and energy -- the hidden costs of the information revolution
In just a few decades, computing and information technology have come to consume fully 5% of all the energy we use. Now artificial intelligence is accelerating the trend.
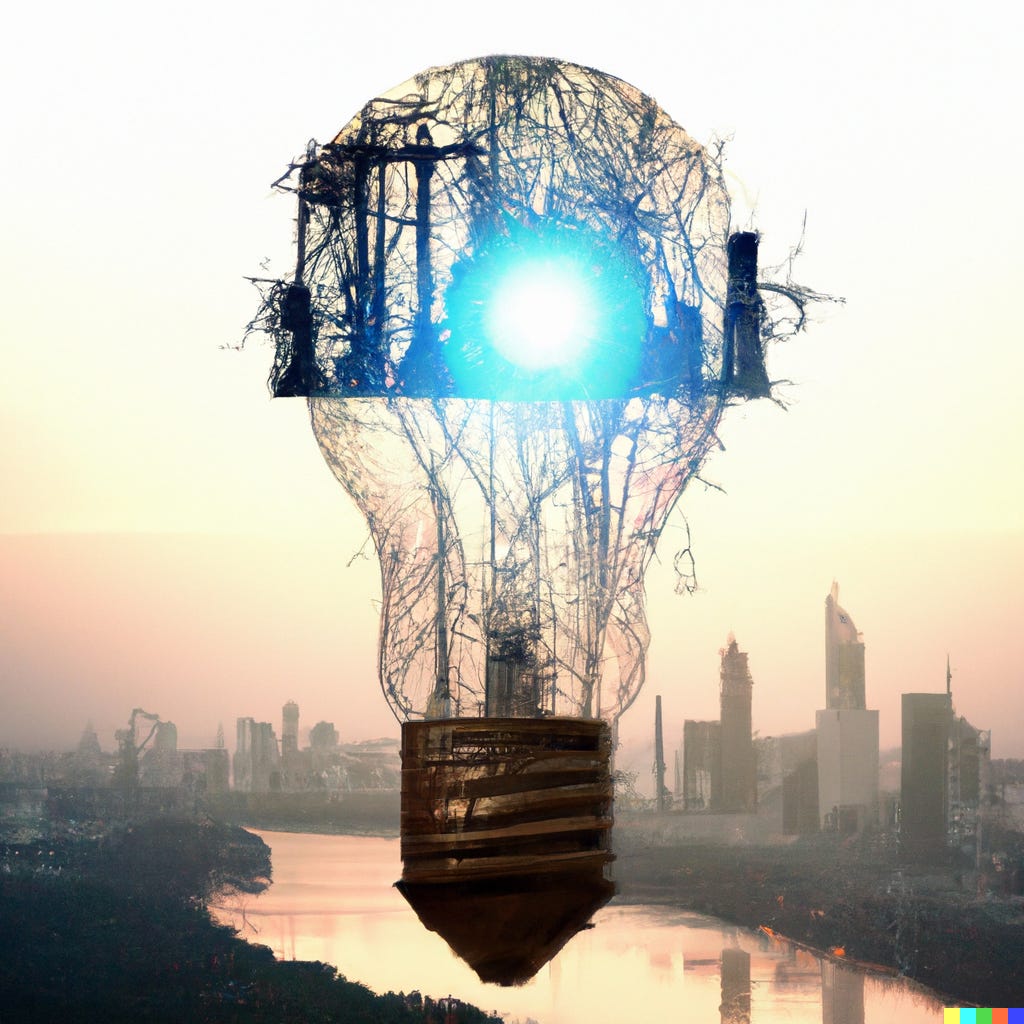
Artificial intelligence (AI) is gradually embedding itself in our lives through services such as real-time language translation or image recognition. The web was on fire last week over uses of large-language algorithms such as GPT-3, which can create disturbingly interesting artwork from short text descriptions, among other uses. Take the image above. After signing up with OpenAI, I used the program called DALL-E to generate this image using nothing more than the text prompt: “ai energy future.” For me, there’s something disturbingly apocalyptic about the image, and there’s far more that comes out compared to what I put in.
Less obvious but perhaps more important uses of AI will be scientific, ranging from the automated discovery of new enzymes for chemistry or AI algorithms making physics simulations run billions of times faster. We should expect more of the same, and probably an acceleration, as AI methods also make it easier to discover better AI methods. One day soon an AI might even begin to break free of human control, with potentially alarming outcomes.
But one thing we can be reasonably sure AI won't break free of are the laws of physics. Computing takes energy, and the algorithms and computing systems of the near-future are going to be using ever more of it. In this respect, AI isn't just amazing in its achievements, but also in its voracious energy appetite. If our future is one of ever more powerful and pervasive computing, then it is probably also one of ever more spectacular consumption of energy.
Our current AI success isn't really based on deep insight into how the human brain works, but on brute force crunching of tremendous volumes of data to tease out subtle statistical relations. As one recent discussion of the issue noted,
The latest language models include billions and even trillions of weights. One popular model, GPT-3, has 175 billion machine learning parameters. It was trained on NVIDIA V100, but researchers have calculated that using A100s would have taken 1,024 GPUs, 34 days and $4.6million to train the model. While energy usage has not been disclosed, it’s estimated that GPT-3 consumed 936 MWh.
For comparison, 936 MWh is roughly the amount of energy used by 100 typical US homes over the span of a full year, or the energy generated by a typical large wind turbine operating over a month.
This is something to ponder. Every time we use the miraculous tools to which we’re increasingly accustomed – speaking a question to google and getting a response, running online language translation, or using AI software to touch up our photos or create images, as I did above — we’re taking part in the use and dissipation of vast amounts of energy.
Of course, hardly anything in our user experience would help us to feel and understand this. And I doubt many companies will be working to make the link more clear.
More concerning yet, the amount of energy we're using with AI computing is increasingly rapidly. As a recent article in The Economist noted, since 2018, the amount of energy used to train large AI models has been doubling every 3.5 months or so. This is evident in the figure below, showing general trends in AI consumption over the past 60 years, with the trend accelerating sharply in recent years:
But let's not just blame AI. What is true of AI is true of computing in general – its energy appetite is always expanding, with the most famous and probably most depressing example being cryptocurrency, and especially bitcoin.
An excellent article last year in the Harvard Business Review looked at various aspects of Bitcoin energy use, and trends in mining bitcoin, which is now often carried out in facilities able to exploit energy resources in remote areas around the globe. This article cited a study by the Cambridge Center for Alternative Finance, which estimates that Bitcoin currently consumes around 110 Terawatt Hours per year — roughly 0.5% of global electricity production, about equal to the annual use of small countries such as Malaysia or Sweden.
Not a huge amount globally, perhaps, but quite a lot for a financial infrastructure that most people see as little more than a high-tech Ponzi scheme.
But growing energy consumption is also a dramatic trend for more ordinary non-Ponzi computing, as all of us now use on a day-to-day basis. Essentially all computing technology has been using ever larger volumes of energy year on year, despite individual devices becoming more energy efficient. The sheer numbers of devices, their complexity, and the number of tasks they carry out more than compensates for improved efficiency.
A report delivered to the U.K. Parliament estimates that computing and information technology as of 2020 consumed 4-6% of global energy use. That report suggested that these technologies are likely to get increasingly efficient in future, but will also come to use ever more energy, despite increasing energy efficiency. This seems likely, in particular, given the widespread hope that a turn toward virtual infrastructure and smart technologies will somehow play a crucial role in helping societies reduce greenhouse gas emissions.
But is this our inevitable future?
Yet it is an important question whether we're locked into this future of ever expanding energy use. Do we have other options? Unconstrained energy growth will, I've argued, eventually lead to serious planetary warming as our waste heat becomes a far bigger problem that greenhouse gas heating is today. This issue will become of urgent importance a little more than a century in the future. We could avoid it, in principle, if we could somehow limit our energy use, keeping it within the bounds of what can be dissipated by the environment without undue damage.
But is that at all plausible? This question isn't simple to answer, because it ultimately depends on human choices, not just physical principles. Two important facts weigh on the question in different directions, and which will win out is not clear.
The more hopeful of the two issues is efficiency. Computing, it is true, cannot be done without using any energy. In physics, a famous result established in the 1960s by IBM physicist Rolf Landauer is that information is ultimately physical and involves unavoidable irreversible steps. As a result, computing always uses up or dissipates away a certain minimum amount of energy.
But this physics limit, while interesting, is today still a long way from being practically important. In principle, there's still lots of room for making our computing technology more energy efficient.
In particular, the human brain works on its own computational principles, utterly unlike artificial computing devices. And it is notoriously energy efficient, using only 10 Watts of power, about that of a very small light bulb. The average laptop uses about five times as much power, despite having far less computing capacity, although it does excel on tasks involving raw computing speed. Presumably, in future, at least some of our computing technology will in many respects grow to be more like biology.
And biologists actually aren't so very far away from growing computing devices like brains. They're already growing rudimentary brain-like systems called neural organoids, which show some limited signs of realistic neural activity. So brain-like energy efficiency might be achieved in computing at some point, and it’s not crazy to believe that our energy use in computing and elsewhere might in principle get gradually more efficient. This means we could do the same things year on year, yet use less energy.
However, there's a problem: humans generally aren’t content to keep doing the same things, but want to do more and different things, and do them faster and better. In the past, this effect – economists call it the rebound effect – has generally meant that improvements in the efficiency of energy use havn't translated into an overall fall in energy consumption. Rising efficiency has always been more than compensated for by multiplying uses of that energy as people have found news things to do with it.
What will the future bring? No one really knows. Presumably we could limit our energy use for a while if we could gradually reduce the overall human population down to the level of 1-3 billion people, which scientists believe is much closer to the realistic carrying capacity of the Earth's ecosystems. But those 1-3 billion people will keep inventing new things to do, new kinds of computing, new bitcoins, new versions of AI, and many things we cannot now even speak of.
Hence, I find it hard to believe that we'll somehow manage to turn off our curiosity, and restrict ourselves to live within a fixed energy use boundary.